Over the last few years, developments in data-driven numerical weather prediction (NWP) based on machine learning have been very fast. A common setup is to use ECMWF’s ERA5 reanalysis to train global models for medium-range forecasting. These work in a similar way to a conventional model, in the sense that they are initialised from an analysis and step forward in time using a model.
Two of these models have been made public, namely Huawei’s Pangu-Weather (PGW hereafter) and NVIDIA’s FourCastNet. In the last few months, ECMWF staff have built infrastructure to run these models. They can now be run from our archived data as initial conditions, with the output saved in standardised formats and connected to our verification tools.
For evaluation purposes, ECMWF has run 10‑day forecasts initialised from our operational analysis, with output every six hours. For upper-air verification scores, such as 500 hPa geopotential height, PGW shows very competitive results compared to high-resolution forecasts (9 km horizontal resolution – HRES) of ECMWF’s Integrated Forecasting System (IFS) in Cycle 47r3, in other words before the recent upgrade to Cycle 48r1. In this article, we will revisit two extreme cases from the past year to examine the ability of the PGW data-driven model to produce forecast extremes.
Storm Eunice in February 2022
Storm Eunice hit north-western Europe on 18 February 2022 and was covered in ECMWF Newsletter No. 171. The cyclone formed on 16 February from a baroclinic wave west of the Azores. It quickly intensified on 17 February before reaching southern Ireland around 18 February 00 UTC. Later that day, the cyclone caused extreme winds over the southern UK.
The PGW model captured the development of the cyclone well, e.g. in the forecast from 16 February 00 UTC, initialised before the cyclogenesis of Eunice. In that forecast, the minimum pressure at 00 UTC on 18 Feb reached 969 hPa in PGW, compared to 978 hPa in IFS-HRES, 974 hPa in the ECMWF analysis and 976 hPa in the ERA5 reanalysis. The progress of the cyclone was somewhat faster in PGW than in IFS-HRES and in reality, and the cyclone reached its most intense stage earlier. Also, in the first image one can see that PGW is missing some of the small-scale structures that are present in IFS-HRES (and in the analysis), such as increased winds along the trough south of the cyclone.

For medium-range forecasts, PGW gave a clear indication of extreme wind 4–5 days in advance, on a similar range as ECMWF forecasts (not shown). However, the maximum wind was somewhat underestimated in PGW for the southern UK and over the English Channel.
This example shows that PGW is capable of producing very intense extra-tropical cyclones, but it can underestimate maximum wind speeds. The latter is possibly the result of a lack of fine-scale structures in the forecasts, due to the training methodology used to create the model.
UK heatwave in July 2022
In the summer of 2022, the UK reached 40°C for the first time on 19 July (see ECMWF Newsletter No. 173). At 12 UTC on that day, the warmest station was London Heathrow Airport with 40.2°C (later 40.3°C was reached in Coningsby, Lincolnshire). Evaluating the 12 UTC temperature for London Heathrow Airport from earlier forecasts, we see that the ensemble forecast (ENS) predicted warmer than normal temperatures more than 15 days before. Around 10 days before the event, ENS became warmer, and from seven days before the event (12 July 12 UTC) and onwards, the ENS mean was above the maximum from the 20‑year model climate for this time of the year. However, compared to the observed 40.2°C, even the shortest-range forecasts before the event fell short, being around 35°C.
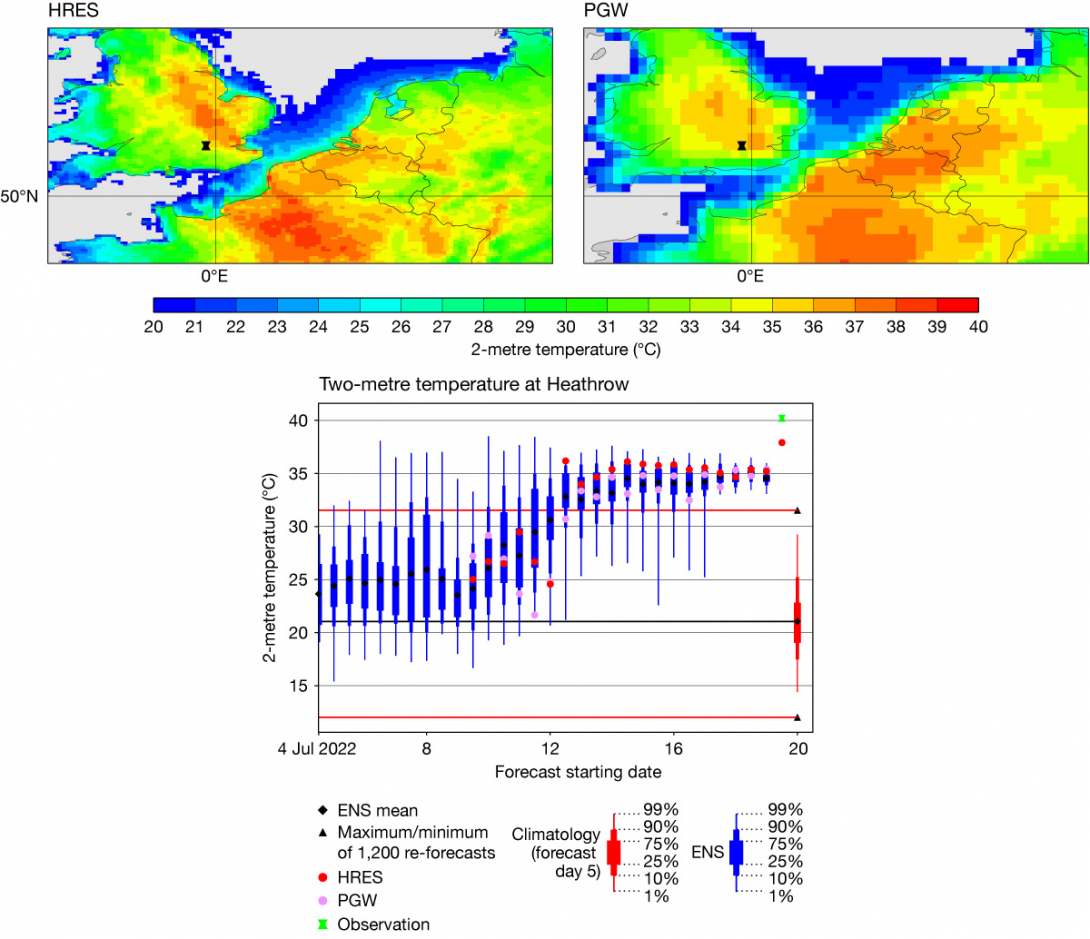
For PGW, although it is a deterministic forecast, the evolution of the predictions followed the trend of the ensemble distribution. From 13 July 00 UTC and onwards, the PGW prediction was above the maximum of the ECMWF model climatology, and for shorter forecasts it was well centred in the ensemble. However, one can note a flip-flopping pattern for PGW, with forecasts initialised at 00 UTC being slightly warmer than those initialised at 12 UTC.
Comparing spatial patterns of 2‑metre temperature in the 1.5‑day forecast, one can see that PGW appears to be smoother than ECMWF HRES, and also to predict lower maxima over both the UK and France, which both had a very extreme heatwave. The smoothing is partly due to lower resolution (about 27 km in the model and the training dataset, compared to 9 km for HRES) but could also be due to the way the model is trained.
Summary
Using cases in ECMWF’s Severe Event Catalogue, we have started to evaluate machine-learning forecasts of extreme cases, alongside statistical evaluation. In this short article, we have looked back at two such cases from 2022 over Europe. The two examples used here show that data-driven models are capable of forecasting extreme weather situations and of providing guidance in the medium range.
The PGW model does not produce forecasts for precipitation, clouds, visibility, wind gusts, etc., which has limited our investigations. Also, there is no reliable ensemble method available yet, due to missing model uncertainty, which is why we have not evaluated that aspect. Future developments of data-driven models are likely to target these shortcomings.
For more details, see the ECMWF science blog on the rise of machine learning in weather forecasting: https://www.ecmwf.int/en/about/media-centre/science-blog/2023/rise-machine-learning-weather-forecasting.