Matthew Chantry, Zied Ben Bouallegue, Linus Magnusson, Michael Maier-Gerber and Jesper Dramsch
Machine learning (ML) has been one of the global topics of discussion this year. Everyone seems to be enjoying the exploration of generative artificial intelligence (AI), in the form of language and image models, to write their boring emails, do their homework or even fix their photos.
At ECMWF, ML techniques for Earth system modelling have been explored for the past few years, for example, using neural networks to better incorporate satellite observations. In 2021 we published a roadmap, trying to do the impossible: predict where the field would go in the next decade. This year we have marked a key milestone in that roadmap: the first operational use of modern ML at ECMWF, using neural networks to monitor observations.
Whilst large language models such as ChatGPT have been dominating the headlines, a quieter revolution has been occurring in the background. ML models are becoming competitive with numerical weather prediction models.
Early exploration of ML forecasting at ECMWF
Our exploration into the topic of making ML-based weather forecasts began in 2018, with ECMWF’s Peter Dueben and Peter Bauer publishing a paper on using ECMWF’s latest reanalysis (ERA5) at around 500 km resolution to predict future 500 hPa geopotential height.
Following on from this came WeatherBench, creating a benchmark problem for ML-based medium-range weather forecasting. Benchmark problems have been key to ML developments in many research areas, providing a dataset and a set of scores. This made the problem very accessible for researchers from a wide variety of backgrounds.
For the next few years, the topic was explored by many authors, but with ML-based models having the equivalent skill of a very coarse-resolution simulation from ECMWF’s Integrated Forecasting System (IFS) (e.g. with around a 200 km grid).
The preliminary conclusion was that this was an interesting research problem to explore, but the likelihood of it becoming operational was low, so it was not a wise investment of ECMWF resources.
A revolution in ML models for weather forecasting
The situation changed rapidly, between February 2022 and April 2023.
In a series of papers, predominantly from large technology companies such as NVIDIA, Huawei and Google DeepMind, rapid progress was made in the quality of ML-based weather forecasts. Currently every few months new contributions are being made to the field.
These ML-based weather forecasts first approached the skill of the IFS (used as the benchmark for high-quality forecasting), then matched IFS skill, and then claimed to surpass our scores. What’s more, making a forecast with these models requires only a single GPU, takes less than a minute, and consumes a tiny fraction of the energy required for an IFS forecast. But is that the whole story?
These fully ML-driven approaches still rely heavily on the IFS. The IFS is used to create both the training and validation data (ERA5), which is essential for any ML model. Moreover, after training, these models rely on the initial conditions from the IFS.
Additionally, the quality of weather forecasts is more than scores, so the question arises: are these ML-based models producing physically consistent and meteorologically meaningful forecasts?
Several of these models have been made public, namely Huawei’s Pangu-Weather and NVIDIA’s FourCastNet. In the last few months, ECMWF staff have built infrastructure to run these models in an easy-to-use pipeline. The models can now be run from our archived data, the output saved in standardised formats, and they can connect to our verification tools. A tool from this work has been released at https://github.com/ecmwf-lab/ai-models for any user to explore the skill of these forecasts.
How skilful are the latest ML-based weather forecasts?
First, the headline scores of the released ML-based models hold up to independent evaluation. When assessed with deterministic scores, such as root mean square error (RMSE) or anomaly correlation coefficient (ACC), Pangu-Weather is a legitimate rival for the IFS (see Figure 1 for example). This holds true not only when assessed against analyses, but also against observations, and when using the same initial condition as the IFS (as opposed to initialising from ERA5, which is done in the public papers).
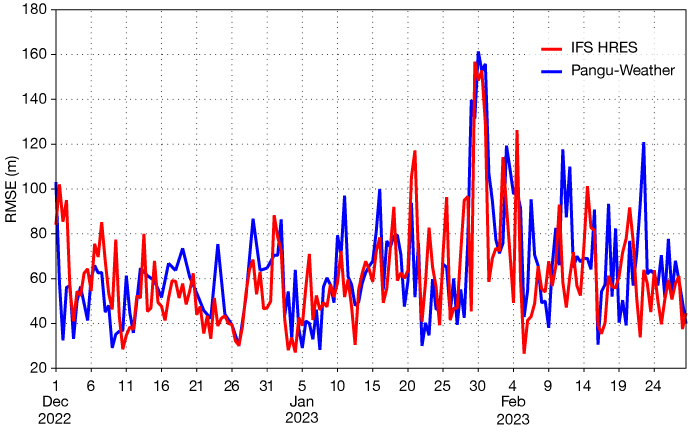
Figure 1: Root mean square error (RMSE) scores of 500 hPa geopotential height for IFS high-resolution forecasts (HRES) and Pangu-Weather over Europe for winter 2022/23 at day 6, measured against operational analysis. Pangu-Weather and the IFS produce comparably accurate forecasts and share a forecast “bust” near the end of January.
However, scores can be optimised, and ML models are trained to do exactly this. Pangu-Weather and FourCastNet were trained to minimise RMSE. Training towards this type of objective can smooth out predictions and it penalises forecasts of extremes. But of course, weather forecasts are at their most valuable for extreme events where lives are at stake.
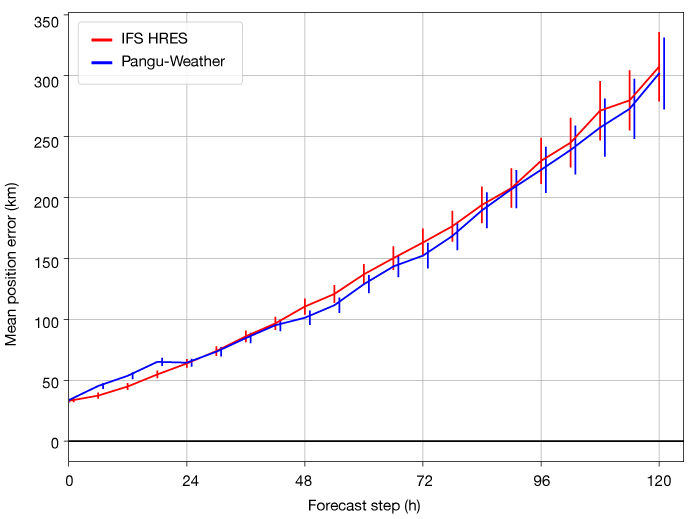
Figure 2: Average tropical cyclone track errors during 2018 for IFS high-resolution forecasts (HRES) and Pangu-Weather, measured against IBTrACS. The statistic is based on events having a tropical storm strength of at least 17m/s, and bars highlight the 95% confidence interval.
Examining the tropical cyclone track accuracy of Pangu-Weather, we see that this ML model performs as well as the IFS model overall within the first five forecast days (Figure 2). The slight advantage for Pangu-Weather after two days is mainly due to reduced along-track errors.
Looking at the case study of tropical cyclone Freddy in 2023 (Figure 3), we also see that whilst the location is well captured by Pangu-Weather (and slightly less accurately by FourCastNet), the cyclone-related winds are considerably less extreme and symmetrical compared with the analysis and the IFS. This is another effect that can be attributed to the training methodology used in the current generation of ML models, by training towards optimising RMSE.
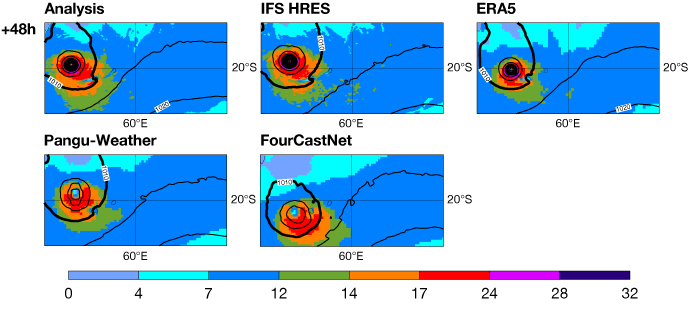
Figure 3: Predictions of tropical cyclone Freddy in 2023 from the IFS HRES, Pangu-Weather and FourCastNet compared with the analysis and ERA5 at day 2. The colour scale denotes wind speed (m/s) and black contours shows mean sea-level pressure. Pangu-Weather and the IFS HRES have very similarly accurate centres, but Pangu-Weather under-predicts wind speed.
Furthermore, evaluation of a range of case studies produces a fairly consistent picture. Data-driven ML models show skill in predicting extreme events (for example, the recent spring heatwave in the Iberian Peninsula), but can lack the intensity predicted by the IFS. This is not a fundamental problem with ML models, but stems from the training methodology. This can be addressed by a range of approaches. For example, adopting a generative modelling approach would encourage more extreme predictions, although it often makes the training of these models a more subtle art.
What is next for ML-based weather forecasting?
In our view, we are currently placed at an exciting moment in weather forecasting history. The minute cost of producing forecasts with these ML-based models means one can envisage building high-resolution ensembles with 500 members instead of 50. Dissemination could possibly be provided by passing an initial condition and a model, allowing users to rapidly run the model and only extract the data of interest to them.
This is in no way the death of conventional modelling. Physically-based models, such as the IFS, have been the key ingredient to generate the ERA5 dataset and initial conditions required to run these ML models. If ML can learn to predict the weather, then it could be deployed in a hybrid sense with physical models to verify, augment and improve the system. These recent ML developments further motivate us to continue our hybrid projects as part of the roadmap.
ECMWF and meteorological centres have unrivalled access to Earth system data and domain expertise, two crucial ingredients in further improving ML models. Now is the time to embrace the technology and establish what is the optimal balance of physical modelling and ML to continue improving forecasts.
We have extra routes to add to our roadmap!
Further reading
You can read more about work at ECMWF on machine learning in weather forecasting in our Annual Report 2022.
Banner image: LV4260 / iStock / Getty Images Plus