
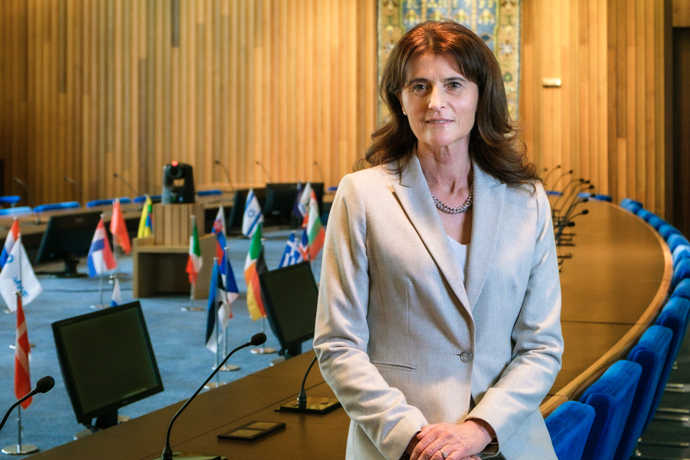
© Stephen Shepherd
An upgrade of the Integrated Forecasting System (IFS) to Cycle 49r1, continued progress on machine-learning forecasts, and work with our Member States on a new Strategy will take place in 2024, ECMWF Director-General Florence Rabier has said. In an interview that previews the Centre’s activities this year, she also highlights other developments in forecasting, research and technology, and she sets out plans for the EU’s two Copernicus services run by ECMWF and the EU’s Destination Earth (DestinE) initiative, in which ECMWF is one of the partners implementing it.
What will be different in 2024 compared to the previous year?
It’s an exciting year because we will develop a new Strategy with our Member States, which will be for 2025–2034. The Strategy will include a much more open vision of what we are going to deliver. This will include, in particular, the development of an optimal way to combine our traditional physical approach to weather forecasting with machine learning. In parallel, this year we are going to make the case for the next high-performance computing facility (HPCF) to be brought in beyond 2027. There are also three collaborative projects with Member States: two have started, on the Internet of Things (IoT) and code adaptation, and a machine-learning project will start this year. This last project is really important as we all face the same challenges and opportunities.
Which main novelties will the IFS upgrade to Cycle 49r1 bring?
The performance of weather parameters will be improved, in particular 2-metre temperature. A lot of changes will take place in parallel: changing the maps characterising the land, land data assimilation developments, and the assimilation of SYNOP weather station observations of 2-metre temperature. Another big development is a new stochastic physics scheme for our ensemble forecasts. This scheme, called Stochastically Perturbed Parametrizations (SPP), helps to create slightly different forecasts to reflect their uncertainty. It has been developed over many years and will become operational in Cycle 49r1. Among many other changes, we also have higher resolution in the ensemble of data assimilations and an improved use of observations.
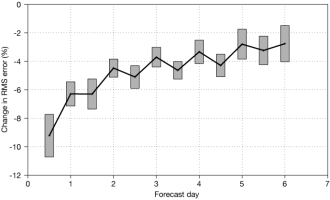
The impact of Cycle 49r1 on 2-metre temperature forecasts verified against SYNOP observations (percentage change in root-mean-square error) for December 2021 to February 2022, 20°–90°N. The grey rectangles show 95% confidence intervals.
Can you give an overview of developments in research?
This year is quite special because many developments for new reanalysis products are converging. Reanalyses represent past ocean, atmospheric composition and weather data. This year, we will for example implement a new ocean reanalysis, ORAS6. Preparations are also under way for a new atmospheric climate reanalysis, ERA6, as well as a new atmospheric composition reanalysis, EAC5. In addition, we are preparing for new ocean and sea-ice models, and for a new seasonal forecasting system, SEAS6. These upgrades only happen every few years, so they are very important. We are also continuing to optimise the use of observations. In particular, there are new and exciting satellite data, for example from EUMETSAT’s first MTG satellite. In terms of model development, we’ll focus on the kilometre scale, and the resolution and the window used in data assimilation will be optimised. Finally, we will test the inclusion of aerosols and atmospheric chemicals in numerical weather prediction.
Which main developments can we expect in forecasts based on machine learning?
We have built an alpha version of the AIFS, and we have released a new version at higher resolution, which is surpassing the systems released by others in terms of forecast scores. We will continue to work on increasing the resolution and improving the method. Very importantly, we are working on how to implement an AIFS ensemble forecast: we’ll make big steps this year even though implementation may have to wait until next year. We’ll continue to work on using the AIFS method in the extended range as well: our Member States are quite keen for us to provide the best possible forecast for week three and beyond. We are also going to progress on the scientific challenge of producing forecasts directly based on observations, using machine learning.
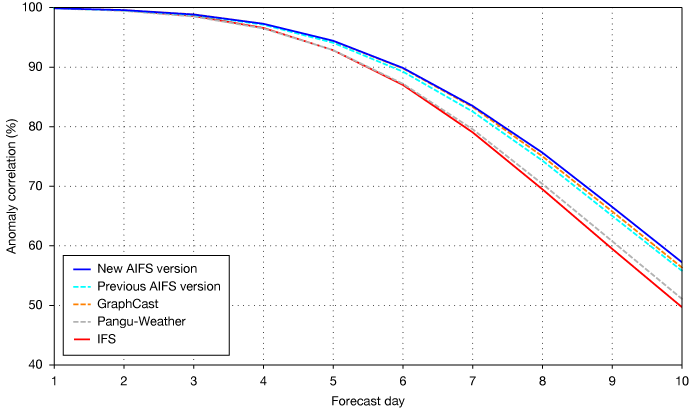
The chart shows the anomaly correlation for 500 hPa geopotential in the northern hemisphere. The latest version of the AIFS has a grid spacing of 28 km, while the previous AIFS version had a grid spacing of 111 km. The graph shows that the new version performs better than other machine-learning forecasts (GraphCast and Pangu-Weather) and ECMWF's IFS.
In which other places is machine learning playing an increasing role?
We will progress on hybrid machine-learning systems, where machine learning is combined with physics-based forecasting and data assimilation to produce optimised results. We’ve already implemented a machine-learning-based observation monitoring system, and there is a nice development of combining machine learning and data assimilation to estimate sea ice concentration. One of the big angles is also how to parametrize model error using machine learning.
What other developments are we working on?
We’ll continue to develop an alternative, nonhydrostatic dynamical core, called FVM. This is to provide additional flexibility at higher horizontal resolutions. We are working on this with our partners in Switzerland. In particular, we will test the FVM running on several multi-GPUs with the GT4Py domain-specific language. Regarding open data, we are going to release quite a number of exciting datasets at 0.25° resolution, which is what we use in the ERA5 reanalysis. In particular, this will be very useful for machine learning. In addition, we are going to provide more data openly for extended-range forecasts, in response to requests from users. For these forecasts, which now run every day, we’ll also implement a new re-forecast configuration, which is an essential component to produce anomaly forecasts.
What big developments can we expect in Copernicus and DestinE?
DestinE, which sets out to create a digital twin of our planet, will see the launch of phase two in June. We have already developed two global twins of Earth, on Climate Change Adaptation and Weather-Induced Extremes, and we are progressing on the On-Demand Weather-Induced Extremes Digital Twin with our partners. The Copernicus Atmosphere Monitoring Service (CAMS) is working on EAC5, and the Copernicus Climate Change Service (C3S) is finalising ERA6. An anthropogenic greenhouse gas emissions Monitoring and Verification Support Capacity (CO2MVS), to be run by CAMS, is being built, and there will be an earlier, joint World Meteorological Organization (WMO) and C3S climate report for 2023. We’ll also be working more closely with EU member states by extending the national collaboration programme for CAMS and implementing it for C3S.
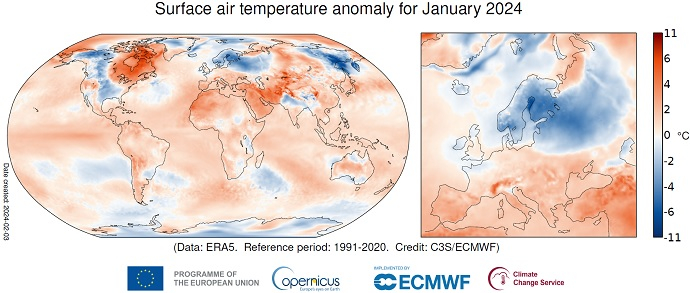
January 2024 was the warmest January on record globally. The maps show the surface air temperature anomaly for January 2024 relative to the January average for the period 1991–2020. Data source: ERA5. Credit: Copernicus Climate Change Service/ECMWF.
What changes are on the cards in technology this year?
In computing, we will continue to make the most of the current HPCF, and the Hybrid2024 project should deliver a benchmark this year for the next HPCF. Around 70–80% of the model is going to be ready this year to prepare for future configurations to be run on GPUs on the next HPCF. We will continue to develop our common cloud infrastructure, migrating the Copernicus Climate and Atmosphere Data Stores into it. There will also be a review of the setup of our new data centre in Bologna after more than a year in operations, optimising resilience and recovery, and we’ll add some GPUs to the current setup, for the code to be adapted and for machine learning. We’ll continue to optimise the sharing of data, for example using a new tool called Polytope, allowing users to extract features more quickly. And finally, we’ll perform a lot of work on migration to the GRIB2 file format.
In a nutshell, what is ECMWF’s aim in 2024?
In 2024, a lot of developments will come together that have been in preparation for several years: a new generation of reanalyses and seasonal forecasts, reaching pre-operational levels for the digital twins of DestinE, and being able to run a large majority of the components of the IFS model on GPU architectures. We will also have a deeper look at the future, which is very open at the moment: we’ll be working on our next Strategy with our Member States, and in particular on the future role of machine learning in weather forecasting.