On 10 January 2024, we introduced a new version of the AIFS. While the previous version had a spatial resolution of 111 km (1°), the revised AIFS version has a resolution of 28 km (0.25°). Its input and output grids are now the native ERA5 reduced Gaussian grid, which provides near-constant resolution across the globe.
There were also architectural changes. The first implementation of the AIFS was built upon Deepmind’s GraphCast approach, based on message-passing graph neural networks and with an internal icosahedral grid with multi-scale edges.
In this new version, the encoder and decoder use attention-based graph neural networks, very similar to a transformer (Vaswani et al., 2017) architecture. The processor now works on an octahedral reduced Gaussian grid, the same kind of grid that is used in our operational IFS. The processor is a transformer that processes the 40,320 grid points of the processor grid as a sequence with a sliding attention window (Figure 1). These layers are highly efficient on GPU architecture, meaning the model is faster both to train and to make predictions.
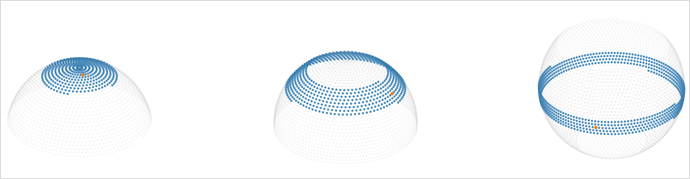
Figure 1: Attention windows (grid points highlighted in blue) for different grid points (red). The grey grid points show an example of how far information can travel within 6 layers of the processor (the AIFS has 16 processor layers in total). For illustration purposes, a lower-resolution grid is shown than that used in the AIFS.
Compared to the ~1° version of the AIFS, we see improved forecast scores for upper-air variables (Figure 2). At the surface, the increased resolution results in significant improvements for surface variables like 10 m wind speed and 2 m temperature (Figure 3).
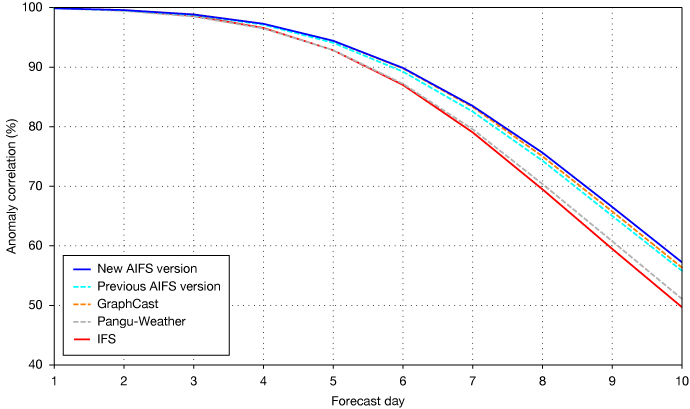
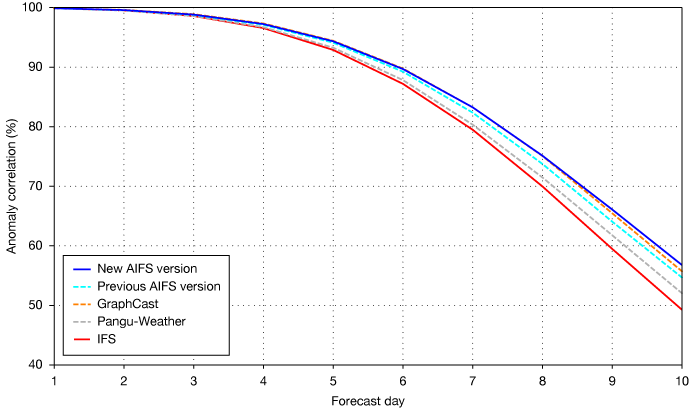
Figure 2: Anomaly correlation for 500 hPa geopotential in the northern hemisphere (top) and southern hemisphere (bottom) for 2022.
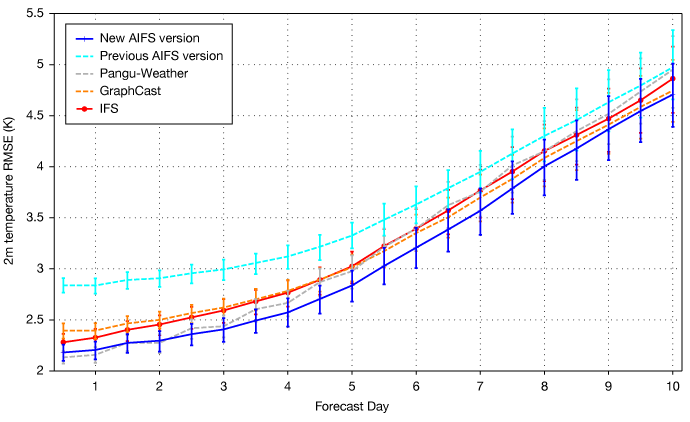
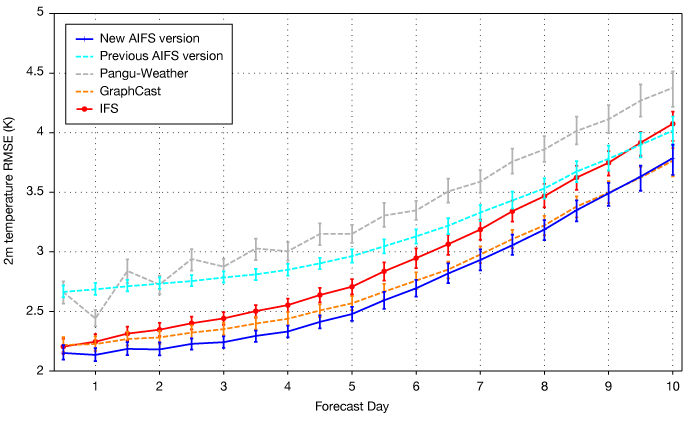
Figure 3: Root-mean-square error for 2 m temperature in the northern hemisphere (top) and southern hemisphere (bottom) measured against synoptic observations for the September–October–November period of 2023. The upgraded AIFS is significantly improved over the previous version, particularly due to the increased spatial resolution.
This work to develop the AIFS is part of two major initiatives approved by ECMWF’s Member States to intensify the development, testing and implementation of machine learning across their weather forecasting chains.
We’ll be back soon with more evaluation of the new AIFS, and updates on precipitation and ensemble prediction.
Authors
Simon Lang and the rest of the AIFS team (Mihai Alexe, Zied Ben Bouallègue, Matthew Chantry, Mariana Clare, Jesper Dramsch, Christian Lessig, Linus Magnusson, Ana Prieto Nemesio, Florian Pinault, Baudouin Raoult, Steffen Tietsche)