Accurate forecasts of two-metre temperature (T2m) are essential for a wide range of applications, including agriculture, energy demand planning and public health. While traditional physics-based numerical weather prediction models such as ECMWF’s Integrated Forecasting System (IFS) still serve as a cornerstone for reliable weather prediction, the emergence of data-driven systems like the newly operational Artificial Intelligence Forecasting System (AIFS) has introduced alternative sources of forecast information. New tools, including the AIFS, require careful evaluation to understand their value to users. This blog post provides a conditional verification of T2m forecasts from the AIFS Single (ML-based deterministic forecasts) and IFS Control forecasts during the winter of 2024/25, focusing on synoptic weather patterns to better understand when and where differences in forecast skill emerge.
AIFS shows notable advantage over Europe compared to IFS
Forecast verification over Europe during the 2024/2025 winter season reveals that the AIFS Single outperformed the IFS in terms of T2m root-mean-square error (RMSE), particularly forecasts for midday (Figure 1, left panel). The most pronounced relative improvements in RMSE during midday appear over flat regions (Figure 1, middle panel), where the AIFS Single delivers over 15% lower RMSE at just 1.5-day lead time. We can note that, over complex terrain, the AIFS Single performs particularly better in the evenings (18 UTC verification time).
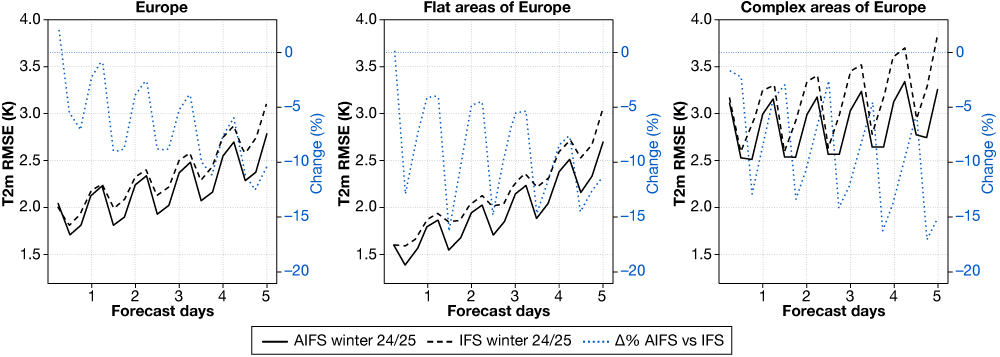
Figure 1: Black curves show two-metre temperature RMSE (K) (lower is better) as a function of lead time for 6-hour time steps for IFS (dashed) and AIFS Single (solid) forecasts initialised at 00 UTC during December, January and February 2024/2025, verified against surface synoptic observations (SYNOP) over Europe (35°–75°N, 12.5°W–42.5°E). Panels show verification against all stations over Europe (left), stations over flat terrain (middle), and stations over complex terrain (right). The dashed blue line denotes the percentage improvement (negative values) of AIFS Single RMSE relative to IFS RMSE.
Several IFS forecasts throughout the winter exhibited considerably larger T2m errors than the AIFS Single forecasts over some regions. One example is the 48 h forecast valid on 31 December 2024 at 12 UTC over south Germany (see boxed region in Figure 2). The AIFS Single forecast aligns closely with observations, showing daytime values mostly below 0°C, while the IFS Control forecast predicts consistently warmer temperatures above freezing.
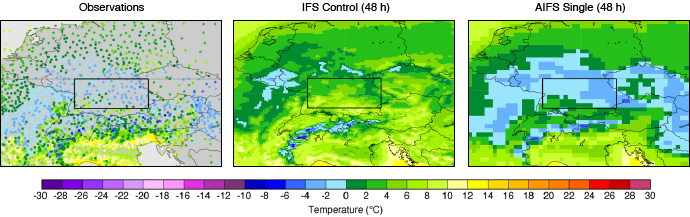
Figure 2: Two-metre temperature (T2m) on 31 December, 12 UTC: Observations (left), IFS Control 48 h (middle), AIFS Single 48 h (right).
The forecast evolution plot (Figure 3) shows that this discrepancy is not unique to the 48 h lead time; it persists across most short-range forecasts (12–96 h). While both the IFS Control and the IFS Ensemble mean overestimate T2m by 2–5°C, the AIFS Single and a prototype of the AIFS-CRPS Ensemble (our experimental ML-based probabilistic forecast) mean stay within 2°C difference of both the observations and the analysis. The contour lines in Figure 4 indicate a high mean sea level pressure (MSLP) system centred over central Europe on that day, which may underpin the skill differences between forecasting systems.
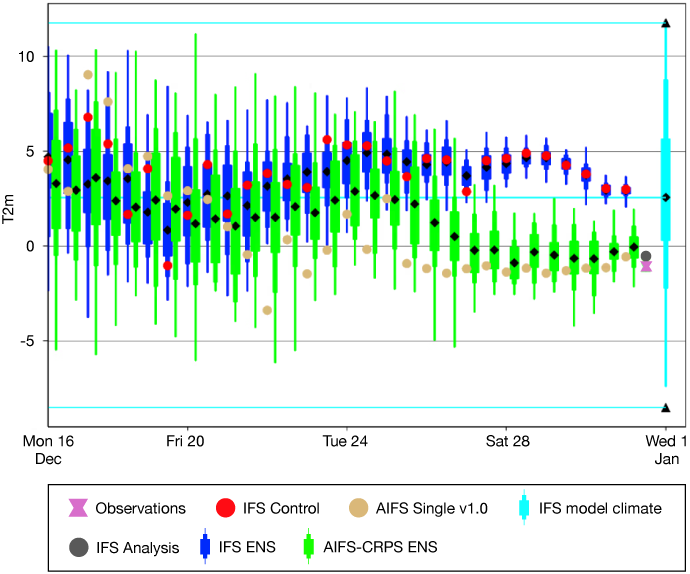
Figure 3: Forecast evolution of T2m over south Germany (black box in Figure 2) initialised from 15 days to 12 hours before 31 December.
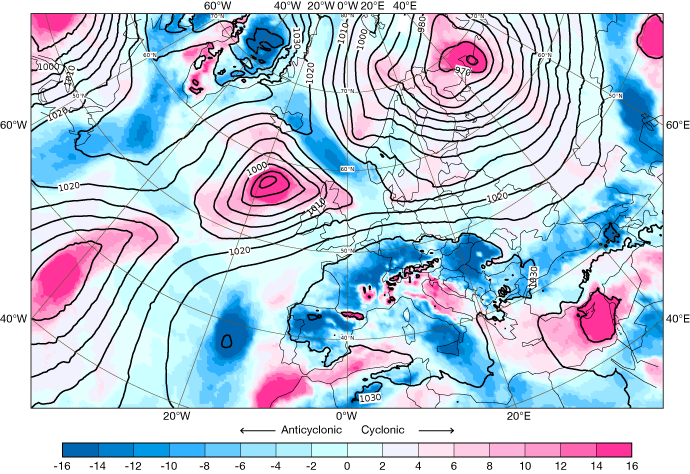
Figure 4: CURV values (shading) and mean sea level pressure (MSLP, contours) over Europe on 31 December.
Conditional verification based on anticyclonic flow over central Europe reveals systematic biases in IFS, but not in AIFS
While the headline scores routinely used at ECMWF for forecast verification provide a general performance overview, conditional verification (e.g. stratified by flow regime) offers additional valuable insight into model behaviour under specific conditions. Daytime warm biases in the IFS during wintertime anticyclonic regimes are a long-standing issue, which is yet to be systematically quantified. During such conditions, low-level clouds often form in the boundary layer below the subsidence inversion caused by the high-pressure system.
To explore connections between the IFS T2m overestimation and the synoptic conditions, we use a new index called the Curvature Using Radial Variation (CURV), a measure of cyclonic or anticyclonic curvature derived from MSLP fields (paper in preparation). CURV values computed from the IFS analysis (AN) over central Europe (44°–55°N, 6°–18°E) show strong anticyclonic conditions over south Germany on 31 December (shading in Figure 4), and such patterns dominated much of the 2024/25 winter (see blue shaded periods in Figure 7).
We use the area averaged CURV values to classify days into cyclonic (mean CURV > 0.5) and anticyclonic (mean CURV < –0.5) and show mean error (bias) maps of the 36 h forecast for each case in Figure 5 and 6, respectively. While there are small differences between the models and the verifying analysis for the cyclonic days (Figure 5), the anticyclonic regime reveals a large T2m bias in the IFS Control (Figure 6). The AIFS Single on the other hand did not suffer from such a large systematic error during this period.
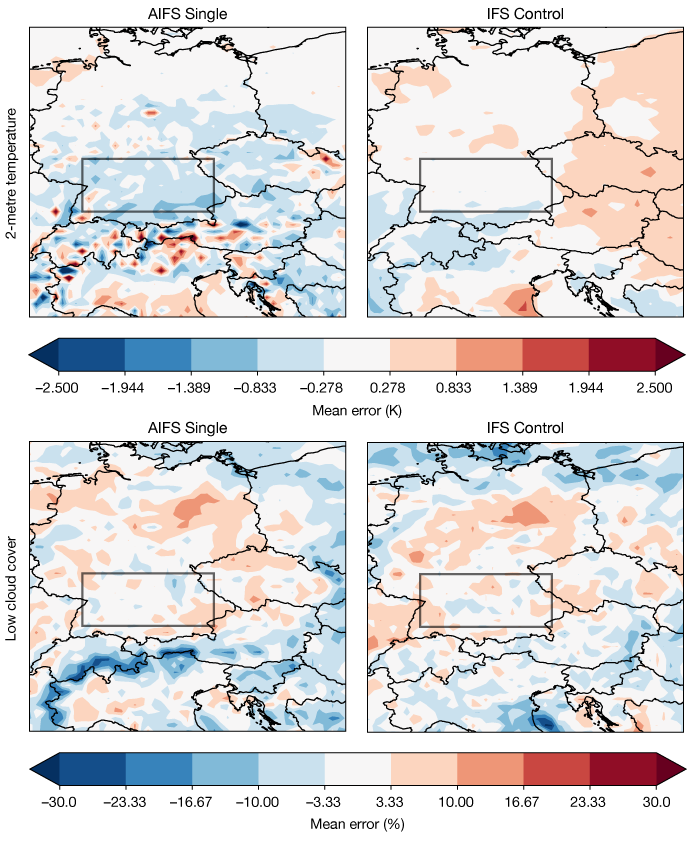
Figure 5: Two-metre temperature (T2m, top) and low cloud cover (LCC, bottom) bias maps for the cyclonic days of December, January and February 2024/2025 from the 36 h AIFS Single (left) and IFS Control (right) forecasts.
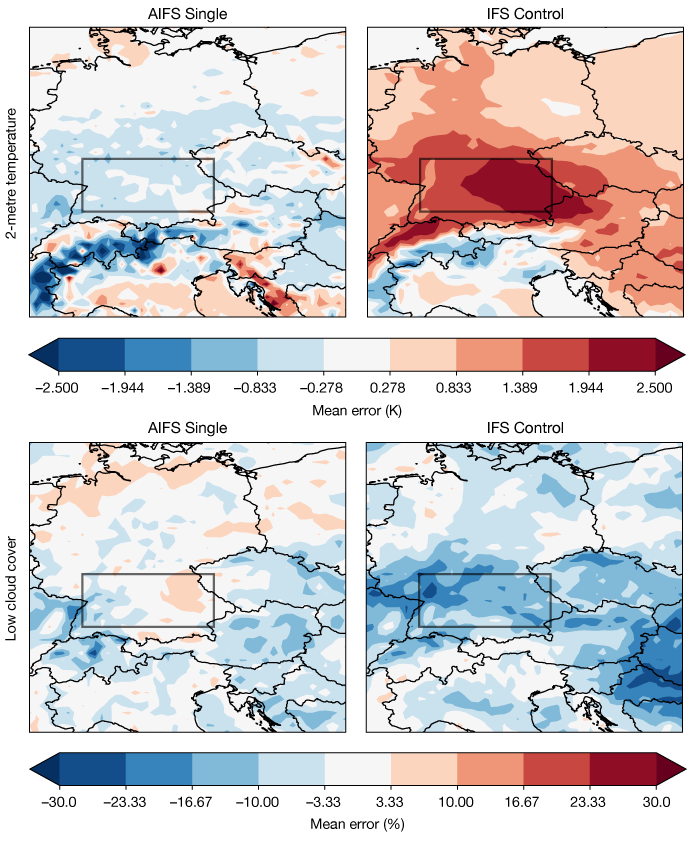
Figure 6. Same as Figure 5 but for the anticyclonic days during December, January and February 2024/2025. Surface temperature errors are closely linked to cloud cover misrepresentation.
Cloud representation plays a key role in the observed T2m biases. The overestimation of daytime surface temperature in the IFS is (physically) consistent with an underestimation of cloud cover during anticyclonic regimes (see right panels in Figure 6). Underrepresenting the cloud cover results in higher short-wave radiation received at the surface and, consequently, temperature forecasts above observed values. Figure 7 presents the three-day running mean of T2m and low cloud cover (LCC) errors over south Germany verified against analysis, showing clearly how temperature errors relate directly to cloud cover errors. The AIFS Single, while also struggling slightly with cloud representation (and hence T2m) during the few days of cyclonic flow in February 2025 (red shaded period in Figure 7), maintains small LCC errors overall during the winter period, which is physically consistent with the smaller T2m errors. Similar results were also found for the IFS verified against SYNOP observations.
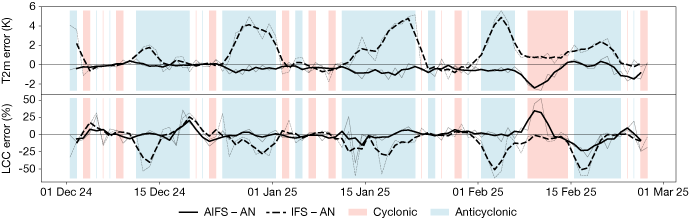
Figure 7: Three-day running mean of area-averaged error of T2m (top) and LCC (bottom) for 36 h AIFS Single (solid) and IFS Control (dashed) forecasts for the south Germany box. Thin lines show area-averaged errors prior to smoothing.
Training and initialisation: Why is the AIFS better near the surface?
These differences in forecast performance are not coincidental, but stem from fundamental distinctions in how the models are built and initialised.
The AIFS Single is trained on ERA5 reanalysis surface temperature, which is constrained by dense observational networks including SYNOP stations, and the model is further fine-tuned on the operational surface analysis. This gives the AIFS Single a strong advantage in representing the connection between surface temperature and its key drivers. In contrast, while IFS initial conditions also incorporate surface observations, the subsequent IFS forecasts rely more heavily on physical parametrizations for surface processes. The representation of low stratus in physics-based models is challenging because of the limited vertical extent of the cloud and the often sharp inversion capping it during anticyclonic conditions. A small overestimation of the turbulent mixing across the inversion may reduce cloud water content and lead to premature breakup of the cloud layer during daytime and an associated overestimation of the diurnal cycle of T2m. These issues are compounded by the major challenge of correctly initialising sharp inversions in physics-based numerical models. Interestingly, upper-level temperature fields such as temperature at 850 hPa do not exhibit similar biases (not shown), confirming that the errors are a boundary-layer issue. Finally, it is worth noting that in both cyclonic and anticyclonic conditions, the IFS Control shows (on average) smaller biases over the Alps compared to the AIFS Single (Figures 5 and 6). This could be linked to the higher spatial resolution in the IFS, which offers a more detailed and more realistic orographic representation.
Summary
This conditional verification analysis demonstrates that the AIFS Single had a clear advantage over the IFS in predicting T2m during this winter’s anticyclonic conditions over central Europe. While both models captured the large-scale circulation well, the large warm daytime errors in the IFS were associated with an under-prediction of low clouds. The AIFS Single captured the T2m much better, and the cloud cover as well. However, more work is required to find the causality of the better prediction by the AIFS Single, i.e. if the better T2m in the AIFS Single is due to better cloud cover.
This short investigation describes a situation where the AIFS Single currently performs better than the IFS. Looking ahead, we will continue to explore how conditional verification can reveal strengths and limitations in both physics-based and machine learning models. Such comparisons may lead to more targeted improvements of the physics-based model. Upcoming blog posts will examine other surface and upper-air variables to better understand the interplay between flow regimes, model architecture, and forecast skill.