
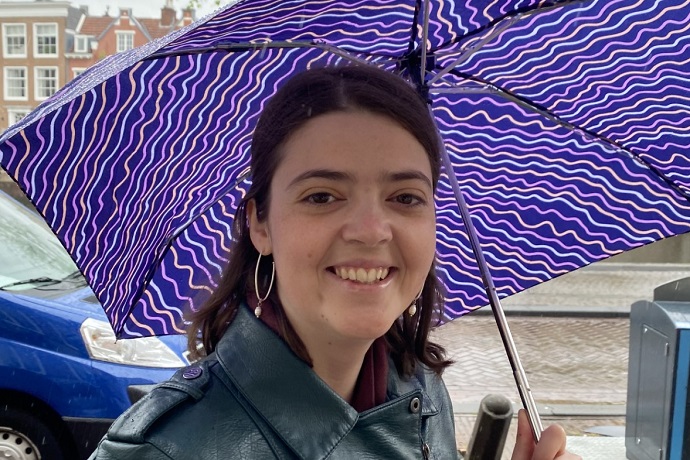
Weather forecasting is experiencing a fundamental shift by adding artificial-intelligence-based models to traditional physics-based models. Mariana Clare is one of the scientists helping ECMWF to make the most of what AI has to offer.
When Mariana studied mathematics at the University of Oxford (UK), she was more interested in applications than in pure maths. One of her A-level subjects had been geography, and she thought she would enjoy a combination of the two.
“What appealed to me was numerical methods, the modelling of fluids, and solving equations which were linked to real-world physics,” she says.
“Soon after my undergraduate and masters course, I decided to pursue a PhD as part of a Mathematics of Planet Earth programme at Imperial College London. I focused on simulating coastal erosion and waves. I liked it because it was a complex numerical problem and a complex fluids problem.”
This animation from Mariana’s PhD shows the mathematical modelling of the erosion of a trench.
The PhD came with an internship at the UK Met Office, where she started to experiment with machine learning for weather prediction. The dataset she was using was based on ECMWF’s ERA5 weather reanalysis.
Mariana pursued the machine-learning theme during another internship at the Institut Pierre-Simon Laplace in Paris, where she studied how neural networks can be used to predict ocean circulation changes, and during a brief postdoc period in London.
Destination Earth
In 2022, Mariana joined ECMWF, initially to work on machine learning for the EU’s Destination Earth (DestinE) initiative. The task was to develop uncertainty information for DestinE forecasts.
“DestinE produces very high-resolution forecasts, which are expensive to run. This makes it difficult to operate a traditional ensemble of forecasts, designed to represent uncertainty. So, I explored using machine learning for this purpose,” she says.
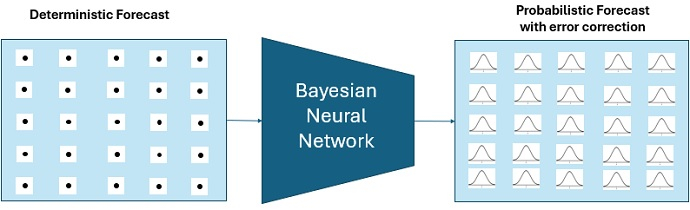
Machine learning can be used to move from a single, deterministic forecast to a probabilistic forecast, which sheds light on how certain the forecast is.
Last summer she switched roles to support ECMWF’s development of a new forecasting system based on machine learning, the AIFS.
What is the AIFS?
Traditionally, weather forecasts are worked out using the laws of physics. The AIFS, on the other hand, establishes a weather forecast on the basis of past developments of the weather. A suitable representation of past weather is for example provided by the ERA5 reanalysis.
Based on a set of conditions from ERA5, machine-learning techniques are applied to distil how the weather is likely to change from a given set of new initial conditions.
“The AIFS is thus fundamentally different from the way weather forecasts have been established in the past,” Mariana says.
Ensemble forecasting using the AIFS
For any forecasting system, it is not sufficient to have a single forecast of the weather. You also need to know how certain, or uncertain, that forecast is.
The answer is traditionally provided by producing a whole ensemble of forecasts. These are based on perturbed initial conditions, and also on slightly different realisations of the forecast model.
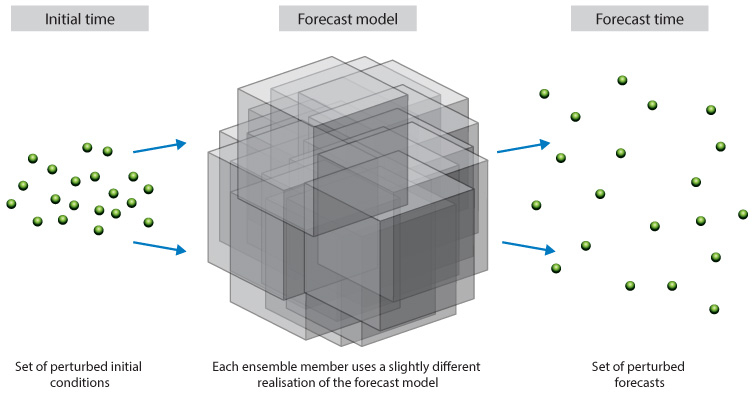
Ensemble forecasts rely on a set of initial conditions that have been slightly perturbed, and on using slightly different realisations of the forecast model.
For the AIFS, the first step is the same: it can use the same perturbed initial conditions as forecasts established using a physical forecast model. This provides about half the desired uncertainty.
But in the second step, there is no physics system that can be slightly perturbed. “We have to learn the uncertainty of the forecast in a different way,” Mariana says. “So, currently we are looking at a method that injects noise into the machine-learning model, and we try to shape that noise in an appropriate way.”
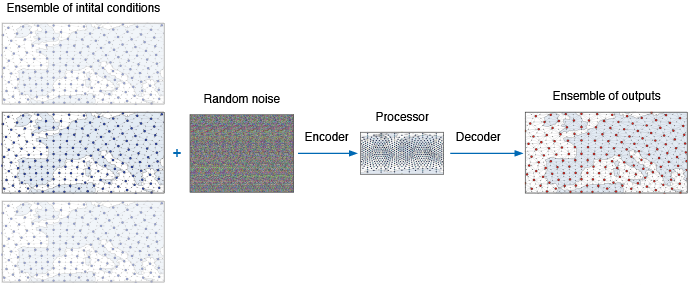
In the current setup of the AIFS, each representation of initial conditions is subjected to random noise that is shaped appropriately to produce a set of ensemble outputs.
“What we have been doing looks promising, but we are still exploring how we can best develop an AIFS ensemble that’s reliable.”
The future of weather forecasting
Forecasts based on machine learning are not only of very high quality, they are also very inexpensive to run in computational terms compared to traditional forecasts.
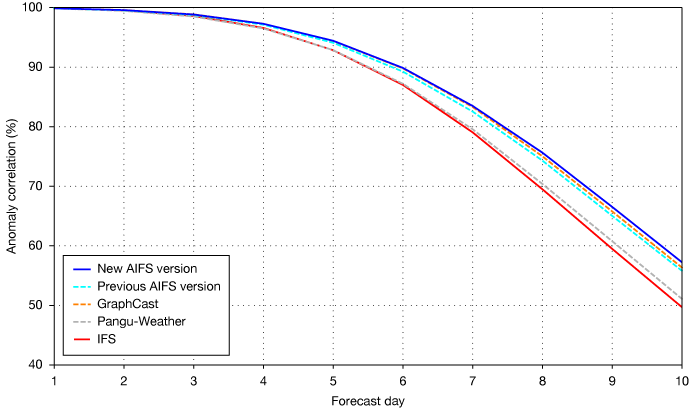
The chart shows the anomaly correlation for 500 hPa geopotential in the northern hemisphere. The latest version of the AIFS has a grid spacing of 30 km, compared to the previous AIFS version with a grid spacing of 111 km. The graph shows that it performs better than other machine-learning forecasts (GraphCast and Pangu-Weather) and ECMWF's IFS.
However, the AIFS does not yet produce the full set of variables produced by the IFS, and it does not yet work at the same resolution. In the medium term, Mariana thinks some changes are likely.
“In the next five to ten years, you can imagine running a traditional forecast at very high resolution with only a very small number of ensemble members, complemented by a machine-learning ensemble with perhaps several hundred ensemble members,” she says.
“There’s definitely a place for the physical model and the machine-learning model to complement each other.”