
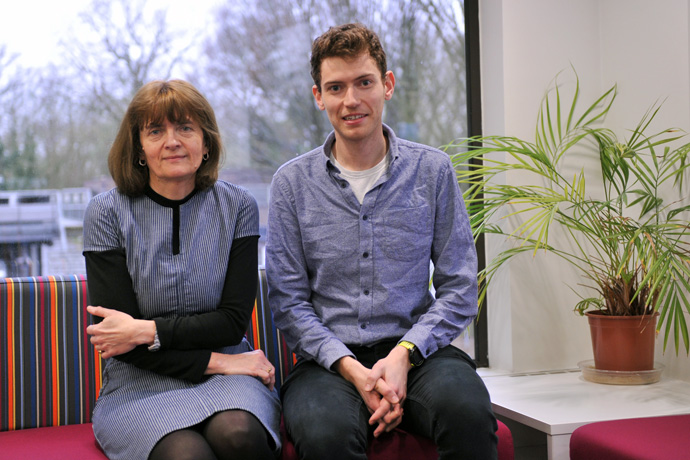
Marta Janisková and Mark Fielding are experts in model physics as well as data assimilation.
ECMWF scientists Marta Janisková and Mark Fielding have demonstrated that using cloud radar and lidar data in operational global numerical weather prediction can improve forecasts. Their ground-breaking results will be presented during the joint NWP SAF/ECMWF/JCSDA workshop on assimilating satellite cloud and precipitation observations for numerical weather prediction taking place at the Centre from 3 to 6 February 2020.
“There were doubts whether assimilating 3-D cloud profile data covering a very narrow band along the satellite’s path would even be possible,” Marta notes. “That is why we are extremely pleased to see that our tests show signs of improvement in forecasts even before extensive tuning has taken place.”
The image shows cross sections of CloudSat radar reflectivity corresponding to various portions of the satellite’s orbital track inside a 12-hour assimilation window for the 00 UTC analysis on 1 August 2007. The data provide information on the vertical structure of clouds.
Preparing ECMWF’s forecasting system for the use of such data to help initialise weather forecasts was a major piece of work, partly funded by ESA.
Marta and Mark’s wide-ranging expertise meant they were well equipped for the task: both studied physics and went on to specialise in numerical weather prediction. Marta did a PhD on developing simplified physics for data assimilation before joining ECMWF in 1999. Mark joined ECMWF in 2016 after completing a master’s in data assimilation and a PhD on remote sensing using cloud radar and lidar.
In addition to working on cloud radar and lidar data, Marta continues to spend half of her time working on simplified physics. This is a critical element of the successful assimilation of observations, especially those sensitive to clouds and precipitation, in ECMWF’s 4D-Var data assimilation system. Mark has also worked on assimilating ground-based weather radar data and on modelling cloud boundaries.
Making the data count
Data assimilation refers to the process of combining Earth system observations with model information to produce the best possible estimate of the current state of the Earth system. This estimate, called the analysis, is then used as the starting point for forecasts.
One of the main difficulties in satellite data assimilation is that generally the instruments used do not directly measure the quantities predicted by forecasting systems.
Cloud radar and lidar instruments are an example of this: they beam radio waves and laser light down into the atmosphere and measure the radar reflectivity and lidar backscatter, respectively.
CloudSat radar observations and CALIPSO lidar observations on 15 September 2009 over the Pacific Ocean and Japan, including a profile view of the eye of Typhon Choi-wan at about 17°N.
The radar signal penetrates clouds and its reflection can thus provide cloud profile information. The lidar signal quickly weakens inside clouds, so the backscatter mainly provides information on cloud top height.
To be able to compare such data with model data, the latter need to be transformed into simulated satellite data using a so-called ‘observation operator’.
ECMWF model data transformed into simulated radar reflectivity and lidar backscatter satellite data for the same day as shown in the preceding image.
“Developing and optimising the observation operator for cloud radar and lidar was a major part of the preparatory work we had to do,” says Mark.
“One of the greatest challenges was to accurately estimate observation operator errors as well as representativeness errors. For example, how representative are the satellite data given their small footprint?”
The work complements existing efforts at ECMWF to better exploit satellite observations of clouds and precipitation in the so-called ‘all-sky’ system. This system has achieved significant success assimilating passive observations (measuring natural emissions from the atmosphere) with sensitivity to cloud and precipitation.
“Radar and lidar are active instruments which measure vertical profiles. There was little previous work to go by because most satellite instruments do not measure such profiles,” Marta notes.
Results
Marta and Mark were richly rewarded for their work when they ran data assimilation tests with cloud radar and lidar data from NASA’s CloudSat and CALIPSO satellites.
They used the full range of regularly assimilated observations at ECMWF to test the impact of adding the radar and lidar data on ten-day forecasts.
“We found valuable improvements in forecast quality across a range of key variables and altitudes,” Marta says.
For example, as shown in the figure below, between forecast days 4 to 8 the error in predictions of temperature at 1,000 hPA was reduced by about 1%. There are improvements for other variables too, but not yet at the same level of confidence.
The charts show the impact from assimilating space-borne cloud radar and lidar observations on forecast errors (root-mean-square error) for a range of variables computed against ECMWF’s own analysis, up to 10 days ahead. The zero line represents errors without the assimilation of cloud radar and lidar observations, so that negative values indicate reduced errors. Bars indicate 95% confidence intervals. All scores are for the whole globe over the period of August to October 2007.
These global figures mask some regional differences. The charts shown below indicate that the biggest reductions in wind forecast errors can be found in the extratropics and at longer time ranges, over a broad range of altitudes (pressure levels).
The charts show the zonal (latitudinal) mean of the difference in root-mean-square error (RMSE) of wind forecasts between an experiment in which cloud radar and lidar data were assimilated and another one (CTR) in which they were not assimilated. The forecasts were verified against the experiments’ own analysis. Negative numbers (blue shading) indicate a reduction in forecast errors resulting from the addition of space-borne radar and lidar observations. Hatching indicates statistical significance at the 95% level. Results are for the period from 1 August to 31 October 2007.
“It is intriguing to see that the biggest impacts are near the poles, which could be due to the scale and structure of clouds there,” says Mark.
Outlook
Marta and Mark’s work on cloud radar and lidar data is by no means finished. Their test results were obtained using a previous version of ECMWF’s Integrated Forecasting System, IFS Cycle 43r1. The next step will be to transfer the code they developed to the current version, IFS Cycle 46r1.
Further down the line, they will have to assess cloud radar and lidar data from new instruments to be launched as part of the EarthCARE satellite mission run by ESA and JAXA. These are expected to become available from 2022.
“The hope is to use the EarthCARE data operationally after extensive testing and tuning,” says Marta. “The results achieved with historical CloudSat and CALIPSO data are very promising. With additional tuning, we expect the impact of EarthCARE data on our forecasts to be even greater.”
Further information
For more details, read Marta and Mark’s ECMWF Newsletter article on ‘Progress towards assimilating cloud radar and lidar observations’.
For more information on the NWP SAF/ECMWF/JCSDA workshop, visit the workshop page on the ECMWF website. Recordings of the talks will be made available on that page.