
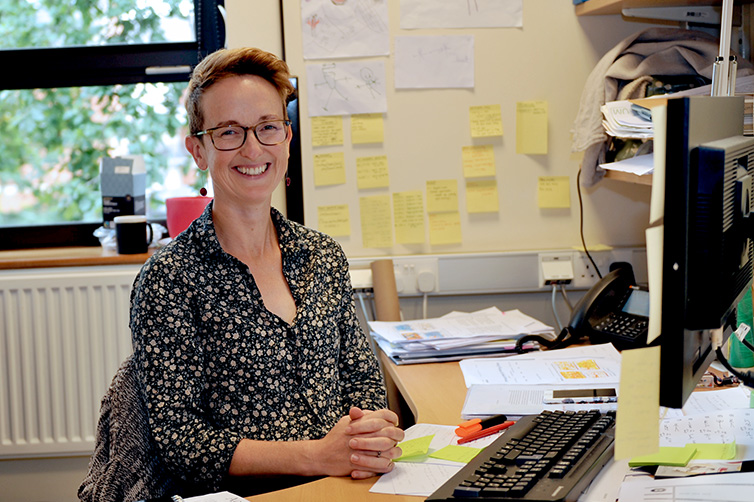
Sarah-Jane Lock’s research aims to optimise methods to represent uncertainty in numerical weather prediction.
How can we best represent uncertainty in weather forecasting models? ECMWF scientist Sarah-Jane Lock will chart the way forward in a talk at the four-day Annual Seminar on ensemble prediction (11 to 14 September).
Dr Lock’s interest in numerical weather prediction (NWP) goes back to her final year as an undergraduate, when she studied fluid dynamics and chaos theory as part of her maths and physics degree course.
She went on to do a PhD in microscale atmospheric dynamics and postdoc work on numerical methods for NWP at the University of Leeds.
This wide range of experience came in handy in 2013, when she joined ECMWF’s Research Department to work on model uncertainty in ensemble forecasts.
“The idea of an ensemble forecast is simple enough,” she says. “Instead of running just one forecast with an unknown error, you run an ensemble of slightly different forecasts. Together they should describe the range of possible scenarios and their likelihood of occurrence.”
“The difficulty lies in constructing the ensemble in such a way that it correctly reflects the uncertainties in our knowledge of the initial state of the Earth system and in the modelling of relevant Earth system processes.”
An ensemble of forecasts produces a range of possible scenarios rather than a single predicted value. The distribution of the ensemble members gives an indication of the likelihood of occurrence of those scenarios.
The sources of model uncertainty
Dr Lock specialises in the uncertainty associated with the modelling of Earth system processes in NWP.
A large part of that uncertainty comes from a set of processes not represented in the equations describing large-scale atmospheric circulation.
Often called the ‘model physics’, these processes are small scale or involve interactions with the atmosphere’s boundaries. Examples include radiation, convection, clouds and turbulent mixing.
Difficulties in the modelling of processes such as radiation, convection and turbulent mixing can introduce uncertainty into weather forecasts.
“The precise effects of some of these processes may not be fully known or they can’t be modelled accurately because our computational resources are limited,” Dr Lock explains.
“For a successful ensemble forecast, we need to be able to correctly represent the uncertainty associated with the model physics.”
How it’s done at ECMWF
Model physics schemes apply adjustments (called ‘tendencies’) to the variables temperature, humidity and wind that are used in the equations describing atmospheric circulation.
ECMWF’s Integrated Forecasting System (IFS) represents uncertainty in the model physics by perturbing the physics tendencies, introducing different perturbations for each ensemble member.
To model uncertainty in the forecasting system, each ensemble member uses a slightly different stochastic realisation of the model as well as a slightly different set of initial conditions.
The tendencies are perturbed randomly within certain limits. However, there is a system to the randomness.
“We have to make sure that each ensemble member represents a credible individual forecast and that, used together, the collection of ensemble members describe a realistic distribution of possible forecast outcomes. This is achieved by using time- and spatially-varying patterns of random numbers to provide the perturbations,” says Dr Lock.
Global evolving patterns of random numbers that include spatial and temporal correlation scales provide the stochastic perturbations for an ensemble member.
Impact on forecasts
The model perturbation method used at ECMWF, known as the Stochastically Perturbed Parametrization Tendencies (SPPT) scheme, produces much-improved forecasts.
In a good ensemble forecast, the spread of the ensemble members should on average correspond to the error of the ensemble mean. This ensures that the forecast is reliable.
It means that, when an ensemble forecast predicts a probability of say 80% for an event to occur, it really will occur in 80% of cases when such a forecast is made. Getting the spread right is thus vital for a successful forecast.
Applying the SPPT scheme leads to a much better spread-error relationship than just including perturbed initial conditions. The effect on forecast skill is particularly pronounced in the tropics.
The Continuous Ranked Probability Score (CRPS) measures how closely the probability distributions of predicted weather events match observed outcomes. The lower it is, the better the forecast. The graph shows the reduction in CRPS for an experiment with SPPT compared to one without SPPT for zonal (east–west) winds at a height of 200 hPa in the tropics (m/s). Results are based on 46 cases during 2015 using 20-member ensembles. Bars indicate confidence intervals at the 95% confidence level.
What next for model uncertainty?
Despite the proven value of SPPT, there is scope for further improvements.
“In some respects SPPT is a rather broad-brush method. For example, at any given time and location, the same random perturbation is applied to all tendencies produced by the model physics,” Dr Lock says.
“While consistency is desirable, the real uncertainty associated with each tendency can be expected to be more complex.”
In SPPT the perturbations are also tapered off near the surface and near the stratosphere. “This is justified for some of the model physics but ignores some sources of uncertainty.”
A radically different approach currently under investigation is called Stochastically Perturbed Parametrizations (SPP).
“SPP still uses random numbers and random patterns but applies them directly to quantities inside the different model physics schemes to get closer to the sources of uncertainty,” Dr Lock says. “This approach gives us much more flexibility than SPPT and makes it possible to improve the physical consistency of the perturbed tendencies.”
Other avenues of research include representing uncertainties in the modelling of the large-scale atmospheric dynamics and of coupled Earth system processes.
Uncertainties associated with the modelling of atmospheric dynamics and of coupled processes, such as the interactions between the atmosphere and the land surface or the ocean, are currently not represented in ECMWF ensemble forecasts.
Optimising ensemble configurations
Other issues to be discussed at the Annual Seminar include ensemble forecasting using limited-area models, seasonal-range forecasting, the predictability of the atmosphere, diagnostics and verification, and optimising ensemble configurations.
For example, how does forecast quality vary with resolution, the number of ensemble members and lead time?
The latter issue is of particular relevance to ECMWF since the Centre’s Strategy 2016–2025 calls for global ensemble forecasts to be run on a 5 km grid.
Numerical weather prediction covers the Earth in a grid and makes forecasts for each grid point. ECMWF’s Strategy includes the ambitious goal of a global ensemble with a 5 km grid spacing by 2025.
The current grid spacing is 18 km up to forecast day 15, so this is a very ambitious goal, not least in terms of the required computational resources.
“Initial tests suggest that for shorter-range forecasts and small-scale weather features resolution is the primary factor in determining forecast quality, while for longer-range forecasts the number of ensemble members becomes an important factor,” Dr Lock says.
“However, this is an active area of research and we do not yet have the full answers. The Annual Seminar will be a good opportunity to catch a glimpse of what the future may hold in this area and in many others related to ensemble forecasting.”