
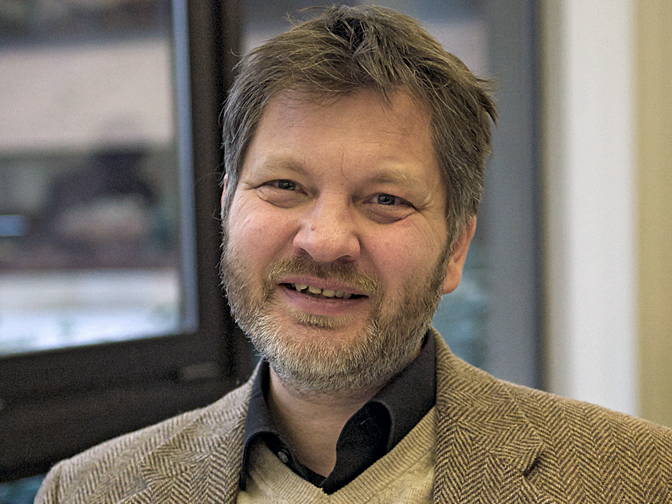
ECMWF Fellow Professor Tilmann Gneiting visited the Centre from 11 to 13 February to discuss recent research into statistical post-processing for numerical weather forecasts. Tilmann leads the Computational Statistics Group at Heidelberg Institute for Theoretical Studies (HITS) and is Professor of Computational Statistics at Karlsruhe Institute of Technology (KIT) in Germany. A mathematical statistician by training, his interest in statistical post-processing for ensembles and probabilistic forecasting started in the early 2000s at the University of Washington in Seattle, USA.
Tilmann’s well-attended seminar on 11 February reported on joint work by HITS and ECMWF. Their research has focussed on the benefits of statistical post-processing (SPP) for ECMWF’s numerical weather forecasts, with the exciting discovery that the benefits of SPP stay about the same even as the raw forecasts improve. In this interview, Tilmann reflects on the challenges and potential of SPP for numerical weather prediction and how his ECMWF Fellowship is serving to intensify collaboration between HITS and ECMWF.
How important is it to properly describe the uncertainty that is inevitable in any weather forecast?
Probabilistic forecasts are important because we need to make decisions based on them. If we don’t properly account for the uncertainty in our forecasts, we can be grossly misled and are bound to make inferior decisions – decisions such as whether an airplane should fly despite potentially adverse weather conditions. We want to calibrate probabilistic forecasts so that what actually happens is compatible with the probabilities stated. The challenge is this: How do we obtain reliable probabilities that are simultaneously “sharp” – as close to 0 or 1 as possible – so that they add value for decision-making? ECMWF is making huge contributions to making those probabilities sharper.
ECMWF produces one high-resolution forecast, a lower-resolution “control” forecast and 50 “perturbed” forecasts. Is it possible to combine these to produce a single overall view of the likelihood of the future weather – can they be considered to be all part of one 52-member ensemble?
The ensemble has 50 members that are essentially indistinguishable from each other and 2 special ones, the high-resolution forecast and the control run. Obviously, treating all 52 members the same doesn’t make sense. We can give the 2 special members a higher weight than the others, but how much more exactly is not so obvious. That’s where statistical modelling comes in, with the aim of getting the most out of the NWP efforts at ECMWF.
Some of the recommendations I made in a Technical Memorandum have been implemented in joint work between ECMWF and HITS.
Can statistical post-processing of the ECMWF forecasts provide more skilful products to forecast users?
Yes, the question is how much more skilful? Often when meteorologists talk about being more skilful, they might say, for example, that we have gained 2 days. What does this mean? It means, e.g., that with post-processing, forecasts 6 days ahead might be as skilful as 4 days ahead without post-processing, and that’s the order of magnitude in improvement we are dealing with when we think about forecasts for specific locations.
A pilot study initiated when my then post-doc Michael Scheuerer visited ECMWF about 2 years ago has identified considerable gains for surface variables, mostly temperature, wind speed, and cloud cover. While post-processing is good for precipitation as well, the gains are not so pronounced as for other variables. This is something we discussed during this visit and which is still a bit of a mystery to us.
As the forecasting system improves, do you expect such post-processing to be still useful in the future?
Yes. This is a question my PhD student Stephan Hemri and a former post-doc of mine, Michael Scheuerer, have investigated with colleagues at ECMWF and documented in a Research Letter under the title Trends in the predictive performance of raw ensemble weather forecasts. The raw forecasts being produced get better every year, and SPP makes them even better. But the natural hypothesis is, given that the raw forecasts are improving, maybe in 20 years we will no longer need SPP. Actually we have found the benefits of SPP stay about the same even as the raw forecasts improve. That’s exciting news, because we can reap benefits twice: we can continue to improve the model and the ensemble, and continue to benefit from SPP. This is great news for the quality of the weather forecasts, and will be the basis for further collaboration.
Can statistical post-processing help with early warnings for severe weather events?
Yes, the most important step for early warning of severe weather events is the use of an ensemble. If you run the model 50 times with distinct initial conditions, you have a much better chance of getting early warnings. How does that interact with SPP? That’s an interesting question and was a key topic during the seminar. Are extreme events something that we need to pay particular attention to in SPP? Do we need special techniques for this, or do standard techniques suffice? Statistical post-processing can certainly help, but there is much scope for future work to determine how exactly it interacts with early warnings and forecasts of extreme events.
Typically, statistical post-processing has been applied to single weather elements at a particular location (such as the temperature in Reading). Are there any ways to calibrate spatial fields, or groups of weather variables while still retaining the physical relationships within the original data?
From a scientific perspective, this is probably the key challenge for the statistical post-processing of weather forecasts, and was probably the most discussed topic at the seminar. Thus far we have looked at one weather variable, at one location, at one look-ahead time. For example, we have applied SPP to improve the forecast of tomorrow’s maximum temperature in Reading. Independently from that, we’ve applied the same sort of technique to improve the forecast for the amount of precipitation in Dublin 2 days from now. But now we can’t really think of distinct locations, look-ahead times, and variables separately from each other. If the question is whether an aircraft should start a flight from London to Dublin, we need to look at what happens in those two places and in between. Similarly, if we want to make plans for a container ship to go from here to North America, we can’t just look at the British and North American coasts. We need to look at doing things jointly, possibly for both precipitation and wind speed, over entire geographic regions, and for longer time periods (for 5 days ahead, for instance, if that’s how long the ship takes).
For the past 10 years, we have mostly focussed on one location, one variable, and one look-ahead time. The ensembles generated at ECMWF honour the physical relationships, but if we then do statistical modelling for each variable individually, we destroy those relationships, and that’s highly undesirable.
We need to restore what’s contained in the model output, and better still, go one step further. Models honour physical relationships but are not perfect at doing it. Maybe we can do better, and correct for systematic deficiencies in those relationships by using statistical techniques. That’s a big challenge. My group and others elsewhere have been looking at a type of technique called the “empirical copula” approach, which is computationally cheap and easy to implement. These are techniques that we are only beginning to investigate closely now, and that we will need to develop further over the next 5 to 10 years. My PhD student Kira Feldmann, who also gave a presentation this week, has been working on this problem.
How can ECMWF’s reforecasts be used to improve the forecast products for users?
Reforecasts are a big help in generating larger training sets for the statistical methods that we apply in post-processing. The current model formulation has been used for about a year. The previous model version was different so we shouldn’t be relying on data from those years to apply SPP for today’s forecasts unless we produce reforecasts retrospectively over the past 20 or 30 years with today’s model. This is a relatively recent development and we’ll surely see a lot of uses of reforecast data over the next couple of years.
How does the ECMWF Fellowship Programme affect your collaboration with ECMWF?
Being a Fellow is a great honour for me and has certainly intensified our collaboration. It’s also a great opportunity for my group, several of whom have become involved in the joint work. The Fellowship Programme enables us to demonstrate our research developments in real-world applications. ECMWF is able to take advantage of the latest research results and can help us to identify areas where future research would bring valuable additional benefits. What ECMWF does and what we do in computational statistics is really teamwork, and almost every project is between several people or institutions. The Programme has made it much easier for us to access ECMWF data without needing to go via our national weather service, the DWD. The Programme also makes our collaboration more visible at my home institutions, which is to everyone’s benefit.