
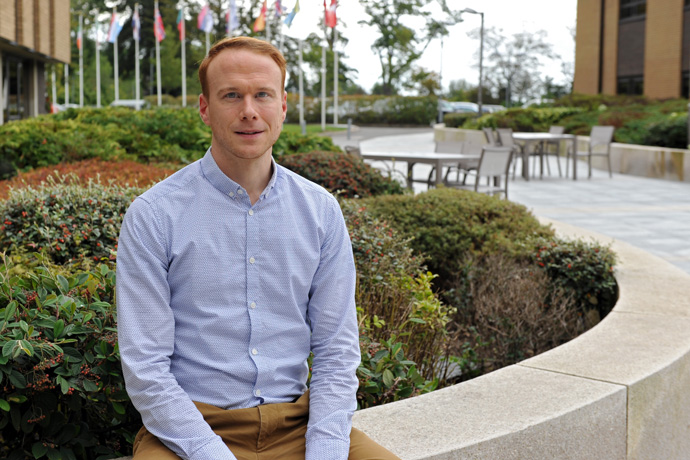
Patrick Laloyaux is one of ECMWF’s data assimilation experts.
Accurate estimates of the current state of the Earth system are crucial for successful weather predictions. ECMWF scientist Patrick Laloyaux has developed a method to reduce the influence of model biases on those estimates.
The state of the Earth system at the start of forecasts is estimated by optimally combining model information with Earth system observations. This highly mathematical procedure is called data assimilation.
The data assimilation system thus calculates a set of initial conditions, called the analysis.
The calculations take into account uncertainties in the model data and observational data, but traditionally they do not account for model bias.
“The new method primarily reduces the influence of temperature biases in the stratosphere on the data assimilation calculations,” Patrick explains.
The stratosphere is the second major layer of the atmosphere between about 20 and 50 km above the surface. The troposphere is where clouds form and weather takes place, but conditions in the stratosphere can influence the weather below.
Temperature biases in the stratosphere have increased in recent years in ECMWF’s Integrated Forecasting System (IFS), so that it became important to reduce their impact on the analysis.
The new method leads to a marked improvement in the quality of the analysis in the stratosphere.
Identifying the problem
Patrick used highly accurate radio occultation satellite data and radiosonde data to review model biases in the short-range forecasts used in the data assimilation system.
He found that there were strong temperature biases of about 0.5°C in the stratosphere, which degraded the analysis.
Successive model changes have led to large temperature biases in parts of the stratosphere in the short-range forecasts used in ECMWF’s data assimilation system. The chart shows how such biases have evolved since 2014 as measured against radio occultation satellite data.
“The use of satellite data covering the entire globe meant that I could analyse the spatial and temporal structure of the biases,” Patrick says.
The results also showed that temperature biases in the stratosphere have increased over the last four years. This is partly the result of the introduction of higher horizontal resolution in the model.
“The impact of higher horizontal resolution on the quality of forecasts has been hugely positive, but an unwanted side effect is an increase in temperature biases in the stratosphere,” Patrick says.
Developing the solution
The method developed by Patrick has a name: it is called ‘weak-constraint data assimilation’. This is not a new research topic, but to date weak-constraint data assimilation has never fully achieved its objective of removing model error in an operational forecasting system.
Traditionally the data assimilation system accepts the model as a ‘strong constraint’ without taking into account possible biases.
The way it works at ECMWF is that the data assimilation system compares the trajectory of a short-range forecast with observations. This is done for many different weather-related variables.
The system then iteratively adjusts the initial conditions of the short-range forecast (the ‘background’) in such a way that differences between the forecast trajectory and observations over space and time are minimised.
The method is called four-dimensional variational data assimilation, or 4D-Var. The process is shown schematically below for a single variable.
In the case illustrated here, for a single parameter x the observations are compared with a short-range forecast starting from a previous analysis xb (the ‘background’) over a twelve-hour assimilation window. The model state xb at the initial time is modified to xa (the new analysis) to achieve a good compromise between model data and observational data within the assimilation window.
When the assumption of a perfect model is relaxed, scientists speak of a weak-constraint data assimilation system.
“In our proposed implementation of weak-constraint data assimilation, the short-range forecast trajectory is adjusted at every time step to correct for large-scale model biases, while small-scale errors are corrected in the background,” says Massimo Bonavita, the Head of the Data Assimilation Methodology Group at ECMWF.
“At this stage, we have mainly tackled temperature biases in the stratosphere, but in principle the method can be extended to other model biases.”
The modified data assimilation process is shown schematically below.
In the weak-constraint formulation of 4D-Var, the short-range forecast trajectory is adjusted by a small amount η at every time step to correct for model biases.
Assessing the results
Having identified the problem and developed a possible solution, Patrick had to assess the impact of the proposed changes.
To this end, he performed experiments in which the data assimilation system was run with the changes (NEW) and without the changes (OLD).
As illustrated below, he found that the method reduces systematic (mean) errors, compared to observations, in short-range temperature forecasts starting from the background (O–B) and from the analysis (O–A).
Vertical profiles of mean errors, compared to radiosonde observations, in short-range temperature forecasts starting from the background (O–B) and from the analysis (O–A) for the currently operational 4D-Var system (OLD) and the new weak-constraint 4D-Var system (NEW). The statistics cover the period 1 October 2018 to 1 May 2019 and show that the new weak-constraint 4D-Var achieves the objective of removing a significant part of the model bias in the analysis.
“These are substantial reductions in errors in the analysis of up to 0.25°C at certain altitudes,” Patrick says. “In addition, we have found improvements in the analysis of tropical stratospheric winds.”
Benefits
While the stratosphere is not where weather takes place, conditions in the stratosphere can influence conditions in the troposphere, particularly at time ranges beyond about two weeks.
“A better analysis of conditions in the stratosphere can be expected to increase the quality of weather forecasts at sub-seasonal to seasonal time ranges,” Patrick says. “The method will also help to reduce biases in the stratosphere in ECMWF’s next reanalysis of past weather and climate.”
The positive test results mean that the version of weak-constraint data assimilation developed by Patrick will be implemented in the next upgrade of the IFS planned for next year.