In a previous Newsletter article (McNally et al., 2024a), we described how ECMWF research teams are embarking on a radical and ambitious project to investigate if weather forecasts can be made directly from meteorological observations, harnessing the power of machine learning (ML). We have called the method Artificial Intelligence–Direct Observation Prediction (AI–DOP). In this issue we report on progress and the first-ever skilful medium-range forecasts made purely from observations alone, without any use of a physics-based model, analyses, or reanalyses.
Here we briefly recall the rationale motivating this research. The initialisation of global physics-based forecast models is extremely challenging. This is because the majority of meteorological observations that we have from weather satellites do not directly measure the variables required by forecast models (e.g. temperature, humidity and wind), and they do not measure at the horizontal and vertical spatial scales required. To address this discrepancy, data assimilation systems blend information from the observations with fine-scale model grid information obtained from a previous (prior) forecast. For this blending process to be optimal, it requires a highly detailed and exacting knowledge of the uncertainty in the observations, as well as the uncertainty in the prior forecast state. As both of these uncertainties can be highly complex and variable (for example changing with the meteorology of the day), specifying these to the degree of accuracy required is extremely challenging and occupies substantial resources. In addition, to successfully blend observations with model states, we need to have a very accurate mapping between the quantities being measured (e.g. radiation being captured by a satellite sensor) and the geophysical variables of the physics-based model state. For some observations, such as cloudy infrared and visible reflectances from satellites, this mapping is so complex that we are currently unable to exploit these data in global numerical weather prediction (NWP).
Using artificial intelligence (AI) technology, we are exploring a completely different approach to using observations. Specifically, we have developed a system to enable Direct Observation Prediction (AI–DOP, see McNally et al., 2024b). Here, by applying ML to long historical datasets of observations, we train a neural network (NN) to forecast how the atmosphere evolves in time. Crucially, this forecast model operates directly upon the physical quantities that are actually measured by our meteorological observing systems. For example, it can predict the time evolution of radiances measured by satellites (that form the bulk of our observations), but also conventional observations of weather parameters, such as two-metre surface temperature or ten-metre wind. Formulated this way, the AI–DOP model can be initialised directly with values from the latest available observations without any need for data assimilation remapping to artificial grids or unmeasured quantities. This obviates the need for estimating large error covariances and allows the use of all observations, irrespective of the complexity of the measurement. The output of the model are predictions of observed quantities at future times. Owing to the design of the neural network and training procedure, the model can produce a forecast at any desired location, even where there may be no real input observations. Forecasts of weather-related variables, such as surface temperature or wind, are obtained by predicting future values of weather parameter observations, such as SYNOP weather station surface data or radiosonde data.
Curation of observation training data
Crucial for AI–DOP is a well-curated set of historical observations that can be used for training. For this, we extract observations from existing operational archives into special data formats suitable for ML training. While this is a laborious process, it is significantly eased by the archives containing standardised data representations (e.g. BUFR), and once this task is complete we anticipate the extracted data will additionally support Member State ML activity via Anemoi, a collaborative, open-source initiative to create ML weather forecasting systems.
At the time of writing this article, we have processed over 250 billion observations from the atmosphere, surface and ocean, covering several decades (some 2.5 TB of data – see Figure 1). This is of course a large volume of data. However, to put these figures into context, the ERA5 reanalysis, which underpins analysis-based data-driven forecast systems like GraphCast and our own Artificial Intelligence Forecasting System (AIFS), amounts to more than 6 PB of data. It is also worth noting that the historical data being curated for AI–DOP are typically at a higher spatial density than ERA5 data, and that they include some observation types which were never used at all by ERA5. The choice of which datasets to prioritise for inclusion in the training was made based on the contribution of each observation type to the current operational 4DVar data assimilation system. During training, the AI–DOP neural network learns statistical correlations between different observation types. A particularly important relationship is that between satellite data (which have excellent global coverage) and sparser in-situ observations of weather parameters. Once correlations are learned at real weather station locations, they can be applied where there are no weather stations (e.g. over oceans) to enable global weather parameter forecasts. If required by users, these forecasts can even be specified on a regular grid.
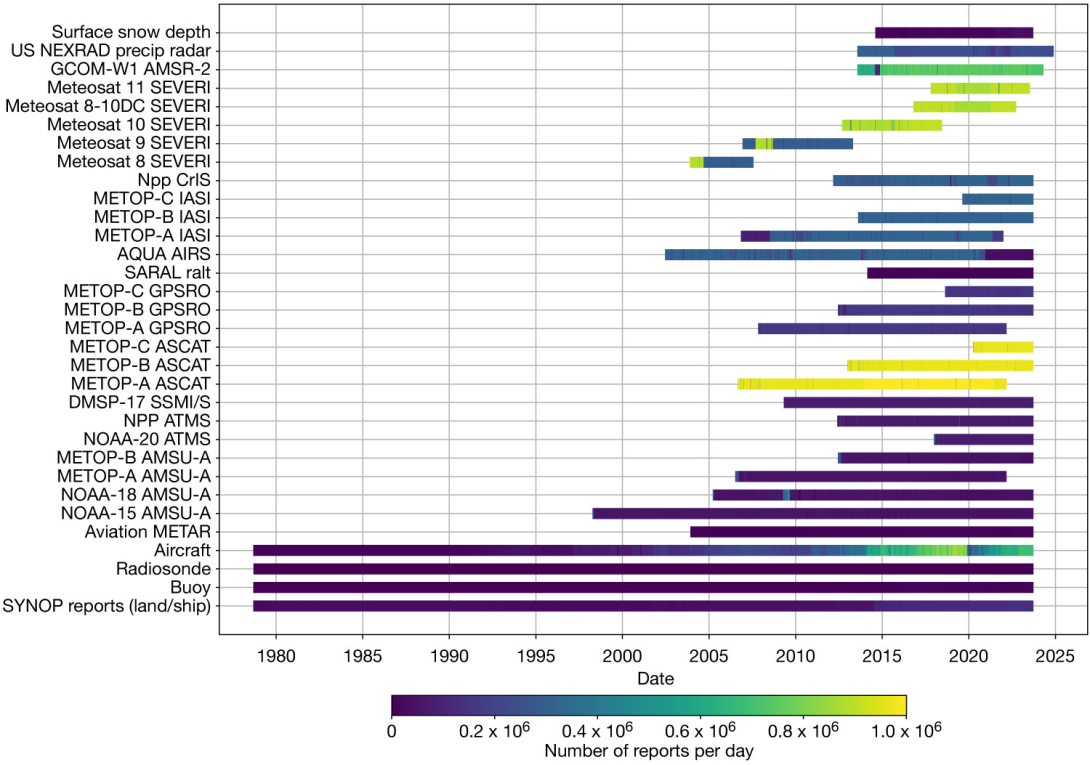
Forecasts using different types of neural networks
We are currently experimenting with two different candidates for the type of neural network to be used in the production of forecasts, a transformer neural network (TNN) and a graph neural network (GNN). While the data curation process is still in progress, we present preliminary results with both networks trained on a subset of observation types. This subset comprises the main satellite-based systems (ATMS, IASI, SEVIRI, AMSUA ASCAT, GPSRO) and insitu conventional observations of weather parameters (from surface stations and balloons).
Both networks are successfully producing predictions of future observations many days in advance, where a highly realistic time evolution of weather patterns can clearly be seen in the predicted values. Both networks have demonstrated the ability to learn robust correlations between global radiance measurements available from satellites and the significantly sparser insitu observations of weather parameters, in order to produce useful weather forecasts. Furthermore, results show that these relationships (once learned at real weather station locations) can successfully be applied to arbitrary locations. In Figure 2, we show an example of predictions projected onto a regular user-specified grid.
Work is continuing to gain more insight into the relative merits of the two different network architectures, with a view to converging upon a single approach (or possibly a hybrid of the two) for further development.
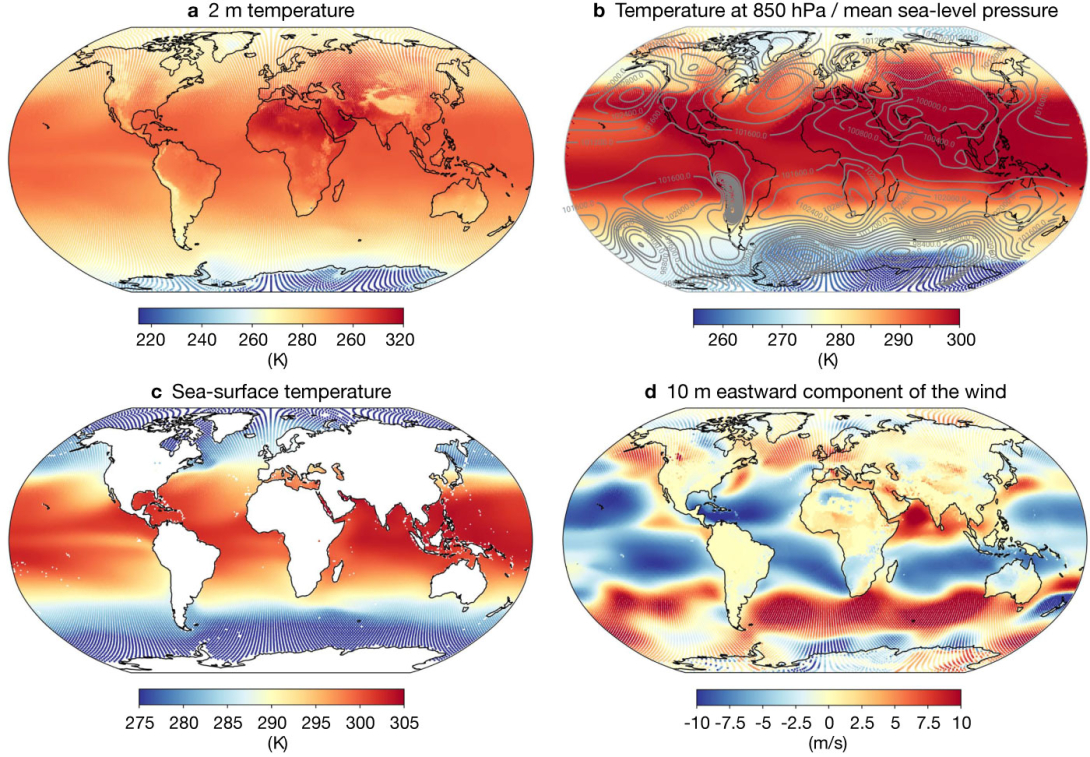
Extending forecasts to the medium range
An immediate priority is optimising the process of forecasting into the medium range. Currently, both the TNN and GNN are trained to take 12 hours of real observations as input and predict observation values 12 hours in the future. To obtain (for example) a five-day forecast, this prediction is repeated 10 times, with the output of one 12-hour prediction fed recursively as the input to the next 12-hour prediction. In Figure 3, we can see that this so-called 'autoregressive roll-out' approach performs extremely well in the short range, but that there is a loss of performance beyond day two. Experience from the development of other data-driven forecast systems suggests that the skill of longer-range forecasts can be improved significantly by fine-tuning the network. This will involve feeding knowledge of the accuracy of the longer forecasts back to the training process to refine the learned correlations. Another area where we hope to achieve accuracy gains is in preferentially learning from tropospheric satellite radiances with a strong predictive correlation to weather parameters (and conversely down-weighting learning of stratospheric data) and guiding the network towards preferentially fitting observations known to be most reliable.
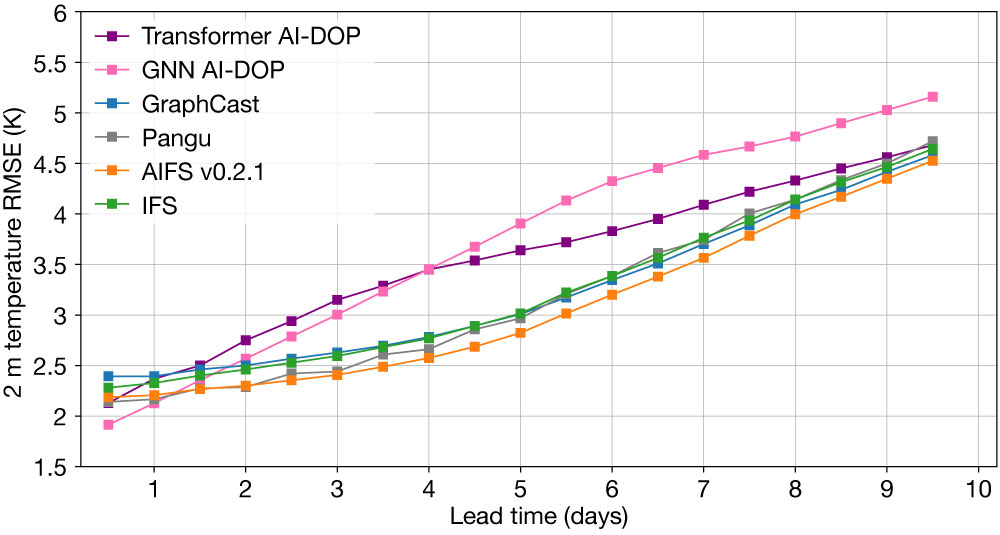
Finally, we are also exploring options for AI–DOP to produce probabilistic forecasts analogous to the ensemble forecasting systems of the physics-based Integrated Forecasting System (IFS) and the AIFS. Here we hope to build upon existing developments, such as diffusion- and score-based models designed for the AIFS (Alexe et al., 2024), which will make it possible to produce ensemble forecasts from AI–DOP. It is also expected that using a diffusion-based model will sharpen meteorological features in the forecast, which are prone to blurring with the current roll-out approach.
Concluding remarks
The successful generation of medium-range weather predictions using only observations is a highly significant milestone in the field of AI data-driven forecasting. AI–DOP represents a radical departure from using observations in data assimilation to create initial conditions for physics-based models or analysis-based data-driven systems. It remains to be seen, of course, to what extent the skill of these new observation-based forecasts, either in the pure form described here or possibly hybridised with other approaches, will challenge other more conventional methods. This activity remains an extremely exciting area of research for ECMWF.
Further reading
McNally, T., C. Lessig, P. Lean, M. Chantry, M. Alexe & S. Lang, 2024a: Red Sky at night... producing weather forecasts directly from observations, ECMWF Newsletter No. 178, 30–34. https://doi.org/10.21957/tmc81jo4c7
McNally, A., C. Lessig, P. Lean, E. Boucher, M. Alexe, E. Pinnington et al., 2024b: Data driven weather forecasts trained and initialised directly from observations. https://doi.org/10.48550/arXiv.2407.15586
Alexe, M., S. Lang, M. Clare, M. Leutbecher, C. Roberts, L. Magnusson et al., 2024: Data-driven ensemble forecasting with the AIFS, ECMWF Newsletter No. 181, 32–37. https://doi.org/10.21957/ma3p95hxe2
Alexe, M., E. Boucher, P. Lean, E. Pinnington, P. Laloyaux, A. McNally et al., 2024: GraphDOP: Towards skilful data-driven medium-range weather forecasts learnt and initialised directly from observations. https://doi.org/10.48550/arXiv.2412.15687