ECMWF is today launching a newborn companion to the IFS (Integrated Forecasting System), the AIFS, our Artificial Intelligence/Integrated Forecasting System (one “I" covering both Intelligence and Integrated).
The AIFS is barely a few months old and proudly entering its alpha version. Its arrival signals the strengthening of ECMWF’s efforts in the field of machine learning (ML), which we have been navigating for a few years now. The AIFS forms one of three components of our new ML project, which began in summer 2023 and aims to expand our applications of machine learning to Earth system modelling.
As our AIFS debuts today with its first outing onto our website, you will be able to view its promising results for yourself and follow our ongoing development. We are scoring it against our long-standing IFS, and you will also be able to check it against the other AI-based models that we are making available on our website on a daily basis.
Physics-based numerical weather prediction models, for us the IFS, are still key in all of this. The IFS is unparalleled by ML models for the breadth of variables it predicts and its spatial resolution. IFS data assimilation provides the live initial conditions and creates training datasets. ECMWF remains firmly committed to further improvement of the IFS.
Our AIFS team explains the choice of technology the system was built on, the resolution of this alpha version, which fields it makes predictions for, and how it compares with the IFS:
What is the AIFS?
In thinking about what technology to build on we considered all the interesting architectures recent papers have developed. Do we want to leverage our knowledge of spectral transforms and build something like NVIDIA’s Neural Operator system, FourCastNet? Do we want to leverage the vast research that goes into vision transformers and build something like Pangu-Weather, FengWu or FuXi? Or do we want to utilise the grid-flexibility and parameter efficiency of Graph Neural Networks like the work of Ryan Keisler or Google Deepmind’s GraphCast?
For this alpha version we have chosen Graph Neural Networks. But we’ve been keen to build a flexible code base so that we can replace any piece of the system if new external or internal developments show promise. Across many aspects, including what data we train on, and how we train the AIFS, we have taken inspiration from all of the previous works referenced above.
Graph Neural Networks allow us to move away from lat-lon grids, which have many points near the poles, and use reduced Gaussian grids which have near equal distance between grid-points no matter where you are on the globe. These are also the grids which are used by the IFS to create ERA5 and operational initial conditions.
This alpha AIFS version has 13 pressure levels, runs at approximately 1 degree resolution and makes predictions for wind, temperature, humidity and geopotential. At the surface AIFS makes predictions for 2 m temperature, 10 m winds, surface pressure and more. We’ll be regularly updating this to increase resolution, and add fields like precipitation.
AIFS was trained to minimise mean squared error, meaning it’s been trained to use as a deterministic model. One of our big next steps, and something we’ll be talking more about in the future, will be around developing an ensemble system. The IFS’s primary offering now is an ensemble prediction system because we know this is the most useful way of making medium-range forecasts.
Here's how we compare with the IFS, for geopotential height at 500 hPa. In meteorology, this is the go-to first measure of extra-tropical large-scale flow, but it is still only a single measure of a forecasting model. More plots can be found on the charts page, which will be expanded as we go. We see that our coarse model is already proving very promising on this metric, which is consistent with results from other ML models.
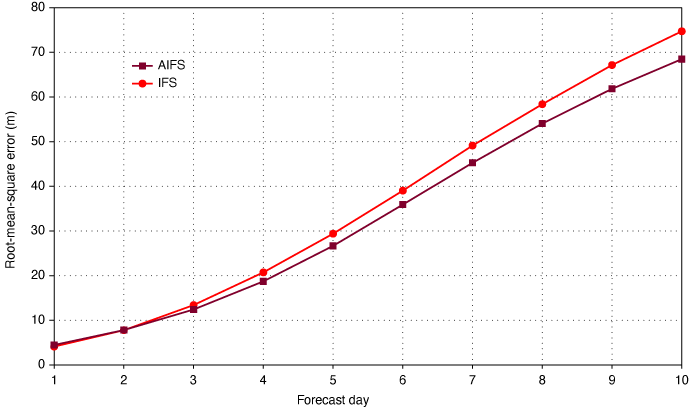
Root-mean-square error in geopotential height at 500 hPa for the IFS and the AIFS in the months of June–July–August 2023 in the northern hemisphere extratropics.
We’ll be writing more in the coming days and weeks, going into more detail on the things that are and aren’t working. We hope you’ll stick around for the journey we are taking…
The AIFS team: Mihai Alexe, Zied Ben Bouallegue, Matthew Chantry, Mariana Clare, Jesper Dramsch, Simon Lang, Christian Lessig, Linus Magnusson, Ana Prieto Nemesio, Florian Pinault, Baudouin Raoult, Steffen Tietsche